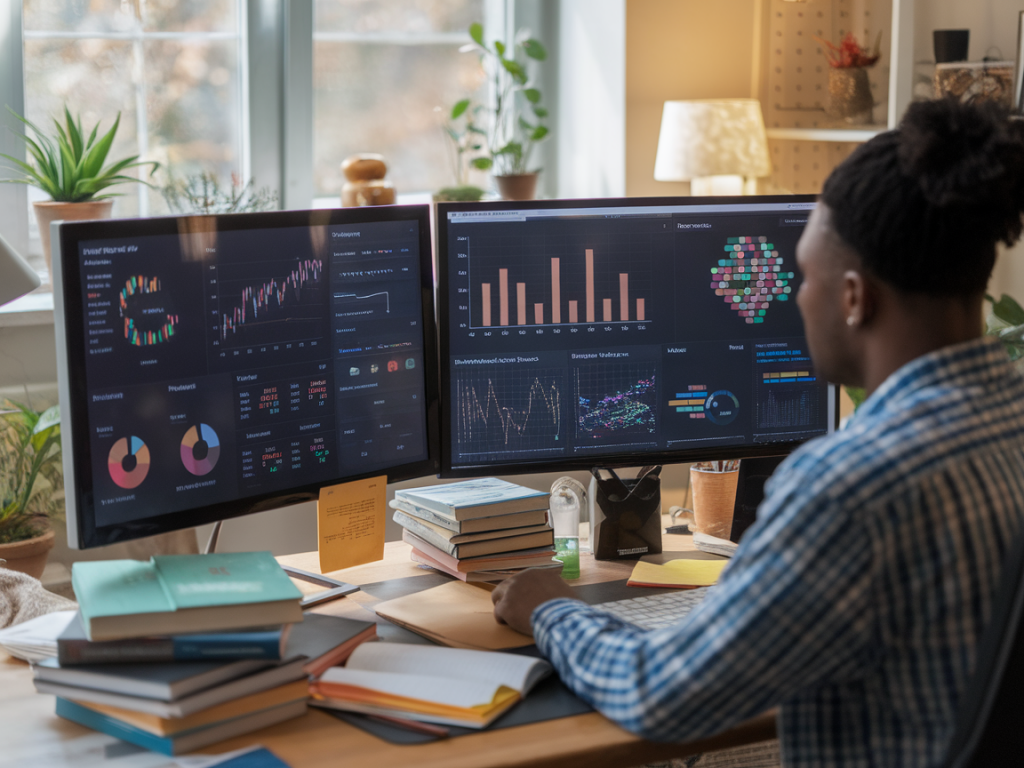
In the dynamic realm of modern marketing, understanding your customers isn't just important—it's everything. In an age where personalization drives success, the true potential of a business lies in unlocking insights around Customer Lifetime Value (CLV). But how do we go beyond basic calculations of CLV? The answer lies in harnessing the power of predictive analytics, a game-changing tool that allows businesses to not only understand a customer’s past behavior but to anticipate their future journey.
Today, I want to dive into how predictive analytics can shed light on hidden opportunities within Customer Lifetime Value. By doing so, you and I can achieve more effective marketing strategies, stronger customer relationships, and, ultimately, a bottom line that grows sustainably.
What Is Customer Lifetime Value and Why Does It Matter?
Before jumping into predictive analytics, let’s revisit CLV itself. In simple terms, CLV represents the total revenue a business can expect to earn from a customer throughout their entire relationship. Think of it as the full potential value of a customer rather than just a snapshot of their current spending.
But here’s the catch: many businesses focus only on immediate performance metrics. They look at monthly sales or acquisition figures without examining the bigger picture of how a client adds value over months or even years. By understanding CLV, you’re able to allocate your marketing resources more effectively, ensuring you’re not just attracting any customer, but the right kind of customer—those who will stay, engage, and purchase long-term.
How Predictive Analytics Revolutionizes CLV
At its core, predictive analytics involves using advanced algorithms and machine learning to forecast future outcomes based on historical data. When applied to CLV, predictive analytics can identify patterns and predict which customers are likely to become loyal, high-value clients—and which are at risk of leaving.
It’s important to remember that CLV isn’t static. For example, a premium customer who currently generates significant revenue might be slowly disengaging. Conversely, a new customer with a low initial purchase may have enormous potential if nurtured correctly. Predictive analytics can help you identify these nuances. Here’s how:
- Segmentation that goes deeper: Traditional customer segmentation often groups clients into categories based on demographics or past purchase behaviors. But predictive analytics layers in additional factors—like spending trends, website behaviors, and engagement rates—to create rich, dynamic clusters. These segments allow for hyper-targeted marketing that speaks directly to future potential.
- Prevention of customer churn: Predictive models can flag early signs of customer attrition, enabling companies to jump in and re-engage customers before it’s too late. For instance, subscription services like Netflix or Spotify use such models to track disengagement (e.g., reduced logins or feature usage) and subsequently launch reactivation campaigns.
- Personalized experiences: By projecting customer needs before they even realize them, brands create hyper-relevant experiences. Amazon, for example, employs predictive analytics to refine product recommendations based on browsing history, purchase habits, and similar user profiles. The more personalized the interaction, the greater the likelihood of unlocking hidden CLV.
Building Predictive Models: Where to Begin?
If you’re ready to dip your toes into predictive analytics, here’s how you can get started, even without a massive data science team at your disposal:
1. Gather and organize your data: Quality data is the foundation of any predictive model. Gather details like purchase history, average order sizes, frequency of purchases, duration of customer relationships, and behavioral metrics (e.g., email click-through rates or app usage).
2. Leverage technology: Depending on your business’s size and budget, there are various tools available for predictive analytics. Platforms like Salesforce Einstein, HubSpot, and SAS Customer Intelligence 360 automatically analyze data and build predictive models. For smaller startups, even services like Google Analytics can provide basic predictive insights using machine learning integrations.
3. Focus on retention and acquisition patterns: Use the data to answer key questions: Which customers are likely to increase their spending? Which ones are showing early signs of disengagement? Which acquisition strategies led to the highest-CLV customers? You’ll begin spotting patterns that guide your decision-making.
Hidden CLV Isn’t Always Financial
One often-overlooked aspect of CLV is the non-financial value customers bring. In today’s social landscape, a customer’s impact goes well beyond their wallet. Here’s what I mean:
- Referral potential: A loyal customer who consistently refers friends can exponentially increase revenue without directly spending more themselves. Think of Tesla’s early referral model, where engaged customers helped build the brand’s cult-like following.
- Social influence: Social media ‘micro-influencers’ can add immense value to your bottom line by showcasing your brand to niche audiences. Many of today’s DTC (direct-to-consumer) brands, such as Glossier or Warby Parker, harness user-generated content to grow organically.
Your predictive models should account for these less tangible contributions to fully realize the holistic value of each customer.
Real-World Success Stories
Let me illustrate the power of predictive analytics in unlocking CLV with two concrete examples:
- Starbucks: Using its loyalty program data, Starbucks predicts customers' next orders and suggests personalized offers through its mobile app. This proactive approach doesn’t just drive one-time purchases—it builds stronger, longer-lasting customer relationships that are reflected in its growing CLV.
- Spotify: By analyzing user data and consumption patterns, Spotify doesn’t just recommend songs—it predicts how engaged a user could become with personalized playlists or premium content. This forward-thinking strategy helps convert free users into paying subscribers consistently.
Bringing Predictive Analytics Into Your Business
While it’s tempting to imagine that predictive analytics requires enormous datasets or costly tools, that isn’t necessarily true for every business. Even small companies can begin analyzing patterns to predict better outcomes. Start simple: look at which products your best customers buy most often, which channels they gravitate toward, and what offers they respond to. Tools like Microsoft Power BI or even Excel can provide actionable insights when used effectively.
As your business grows, so too will the sophistication of your predictive models. While the process can seem overwhelming at first, the rewards far outweigh the initial investment of time and resources. Predictive analytics doesn't just enhance your understanding of CLV—it unlocks vast, hidden opportunities to create deeper connections with your audience.